Minimize AI investment risks with Proof of Concept (PoC)
gaining actionable insights for successful enterprise-wide deployment
potential risks


Delivering Results for Leading
Businesses Across Industries
Tailored AI solutions for scalable innovation and risk mitigation
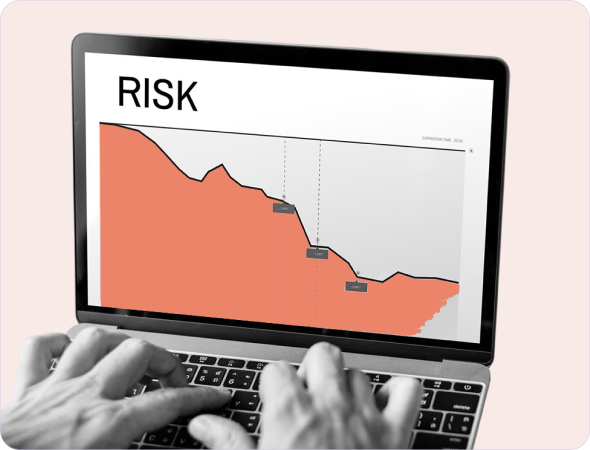
Innovate and reduce risks with real-world prototype development
AI use cases that drive impact
Align stakeholders on KPIs with workshops for high-impact use cases, addressing silos, and achieving operational efficiency and risk reduction.
Set clear KPIs for success
Set clear, measurable performance indicators for your PoC. These KPIs evaluate feasibility, scalability, and alignment with business objectives for informed decision-making.
Build real-world ready prototypes
Build high-fidelity prototypes for real-world use before full-deployment. Torsion ensures each prototype meets enterprise performance standards allowing thorough testing of functionality, security, and integration capabilities.
Test with users, refine for success
Engage real end-users for practical feedback, refining usability and functionality early. This reduces development costs and boosts user adoption.
Prepare your data for scalable, secure AI integration
Bringing data together in real-time
Torsion’s detailed audits for data accuracy and completeness pinpoint improvements in data enhancements, ensuring reliability in AI and LLM models.
Preparing data for ML success
We look at your existing governance practices, suggesting tweaks for better AI-driven data labeling, metadata management, and lineage tracking.
Precision annotation for better models
Our data audit checks your compliance posture with AI/LLM rules like GDPR and CCPA, recommending policy updates and data protection measures.
Privacy and compliance made easy
We comply with GDPR, HIPAA, CCPA, and other regulations, implementing privacy protocols like encryption and anonymization for data protection and regulatory compliance.
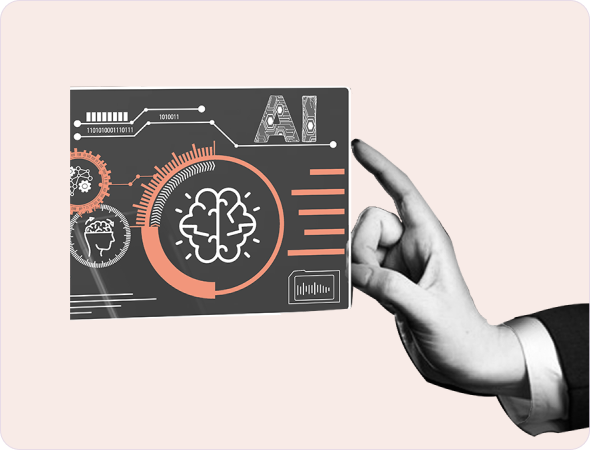
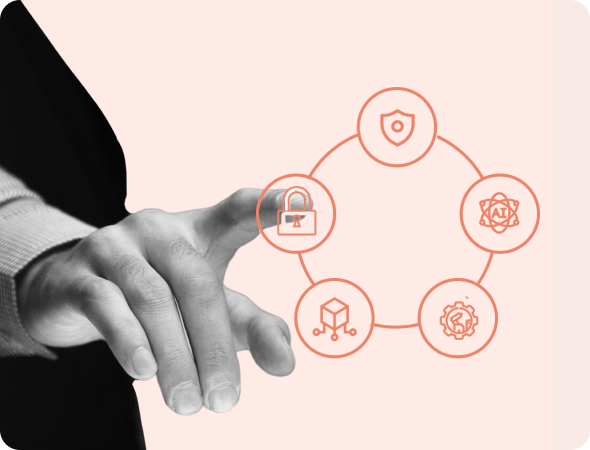
Build custom AI solutions for unique business needs
LLM fine-tuning with domain precision
Torsion builds custom LLMs through supervised fine-tuning with proprietary datasets and reinforcement learning. Using domain-specific data we improve model performance for specialized tasks.
Models built for your industry
We design models tailored to your sector’s needs, whether it’s compliance in finance or real-time processing in retail, ensuring high accuracy.
Advanced performance optimization
Torsion optimizes model efficiency with hyperparameter tuning, model pruning, and GPU/TPU acceleration for maximum speed and accuracy in production environments.
Own your models with open IP
Our experts design modular, API-first architectures, including containerization and microservices, ensuring your tech stack adapts to future business and AI advancements.
Why start with a Proof of Concept (PoC)?
AI
concepts
Operational
risks
Performance
benchmarks
User
feedback
Scalable
implementation
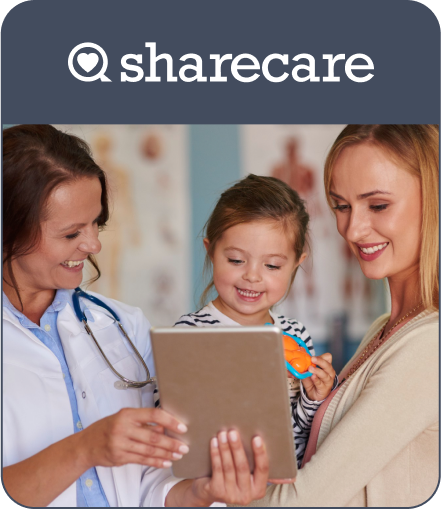


Torsion built an AI-driven care gap analysis system, automating clinical rule application for improved patient insights.
reducing manual review effort


in patient data evaluations
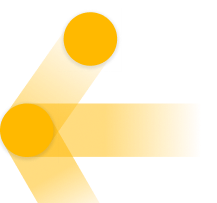
Take the first step toward scalable custom AI solutions
Request a PoC consultationExplore more on enterprise LLM & AI strategy
Your Questions Answered
- How does a PoC support enterprise-wide AI adoption?
A PoC provides a structured environment to test AI feasibility in real-world conditions, allowing Torsion to validate high-impact use cases, establish KPIs, and address integration or compliance challenges early. This process ensures that only the most relevant, scalable solutions move forward, streamlining AI adoption across the enterprise.
- How does a PoC align with business transformation goals?
Torsion’s PoC approach aligns AI initiatives with your business goals from the start. Through strategic use case scoping and tailored KPI setting, each PoC phase targets measurable outcomes, like efficiency gains or enhanced customer experience, that align with your core transformation objectives.
- What are the main challenges in AI transformation for businesses?
Torsion’s PoC framework simulates real-world application scenarios using high-fidelity prototypes and performance benchmarks. This approach identifies any technical or operational challenges, ensuring the AI solution’s feasibility for full-scale deployment while minimizing risks tied to compliance and resource allocation.
- How can a PoC demonstrate AI feasibility before full deployment?
We define specific KPIs, such as model accuracy, processing speed, and data integration efficiency, tailored to each PoC. These benchmarks provide clear, measurable indicators of success, guiding decisions on refining, scaling, or deploying AI models in production.
- What metrics measure the success of a PoC?
During the PoC, Torsion fine-tunes LLMs with domain-specific data, adjusting vocabulary, tone, and accuracy to ensure the models deliver precise, context-aware outputs. This customization is essential for applications requiring industry-specific language, like legal document processing or customer support in specialized sectors.
- How is LLM fine-tuning approached during the PoC stage?
Fine-tuning enables the LLM to perform optimally within your unique business context. By adjusting the model to your specific use cases, Torsion enhances relevance, accuracy, and user satisfaction, ensuring the AI solution aligns with your operational needs from day one.
- What benefits come from fine-tuning an LLM for a PoC?
Torsion evaluates LLMs based on scalability, processing capabilities, and customization potential, selecting models that can support long-term growth. Factors like data handling, compliance alignment, and integration ease are considered to ensure the model adapts as your business evolves.
- How is the right LLM chosen for scalability in a PoC?
Torsion’s PoC for healthcare includes HIPAA-compliant data handling, real-time processing for patient data, and precision in data annotation. This tailored approach ensures that AI solutions meet the sector’s regulatory requirements while optimizing for patient outcomes and operational efficiency.
- What compliance and privacy measures are required for healthcare PoCs?
In healthcare, Torsion’s PoC incorporates data encryption, anonymization, and access controls to ensure compliance with standards like HIPAA and GDPR. These measures protect patient data integrity while allowing healthcare providers to test AI applications safely.
- How does a PoC address compliance with financial regulations like GDPR or CCPA?
For finance, Torsion’s PoC includes stringent data privacy protocols, compliance-driven KPIs, and real-time monitoring to meet regulatory requirements like GDPR and CCPA. This approach minimizes legal risks while supporting secure, scalable AI deployment.
- How is transaction data integrated and secured in finance PoCs?
Torsion ensures secure integration of transaction data by using encryption, secure data pipelines, and real-time monitoring. This protects sensitive information and supports accurate, compliant AI applications in areas like fraud detection and customer segmentation.
- What use cases are prioritized for PoCs in the retail industry?
For retail, Torsion focuses on high-impact use cases like demand forecasting, personalized recommendations, and inventory optimization. Each PoC prioritizes solutions that enhance customer experience and drive operational efficiency, with scalability and ROI as key outcomes.
- How does a PoC reduce risks in AI investment?
Torsion’s PoC framework identifies and mitigates risks early by testing in controlled, real-world conditions. Through iterative prototyping and KPI tracking, we address potential technical, compliance, or operational challenges before they impact full-scale deployment.
- What measurable value does a PoC provide for decision-making?
By setting precise KPIs and benchmarks, Torsion’s PoC offers clear indicators of model performance, scalability, and alignment with business goals. This data-driven approach supports confident, well-informed decisions on AI scaling and resource investment.
- How is the transition from PoC to full-scale implementation supported?
Torsion’s PoC process builds a foundation for scalable AI by validating performance and collecting real-world user feedback. This ensures that the AI model is ready for production, and Torsion’s integration support helps streamline the transition from PoC to live deployment.
- What custom AI model development services are provided during a PoC?
During a PoC, Torsion tailors models through LLM fine-tuning, domain-specific adjustments, and performance optimization. This customization ensures that each model is aligned with your industry’s unique needs and ready for seamless scaling.
- How is domain-specific data used to customize AI models?
Torsion uses domain-specific data to train and fine-tune AI models, adjusting for industry nuances in language, accuracy, and application. This approach improves the model’s relevance, precision, and usability within your operational environment.